Deep Learning
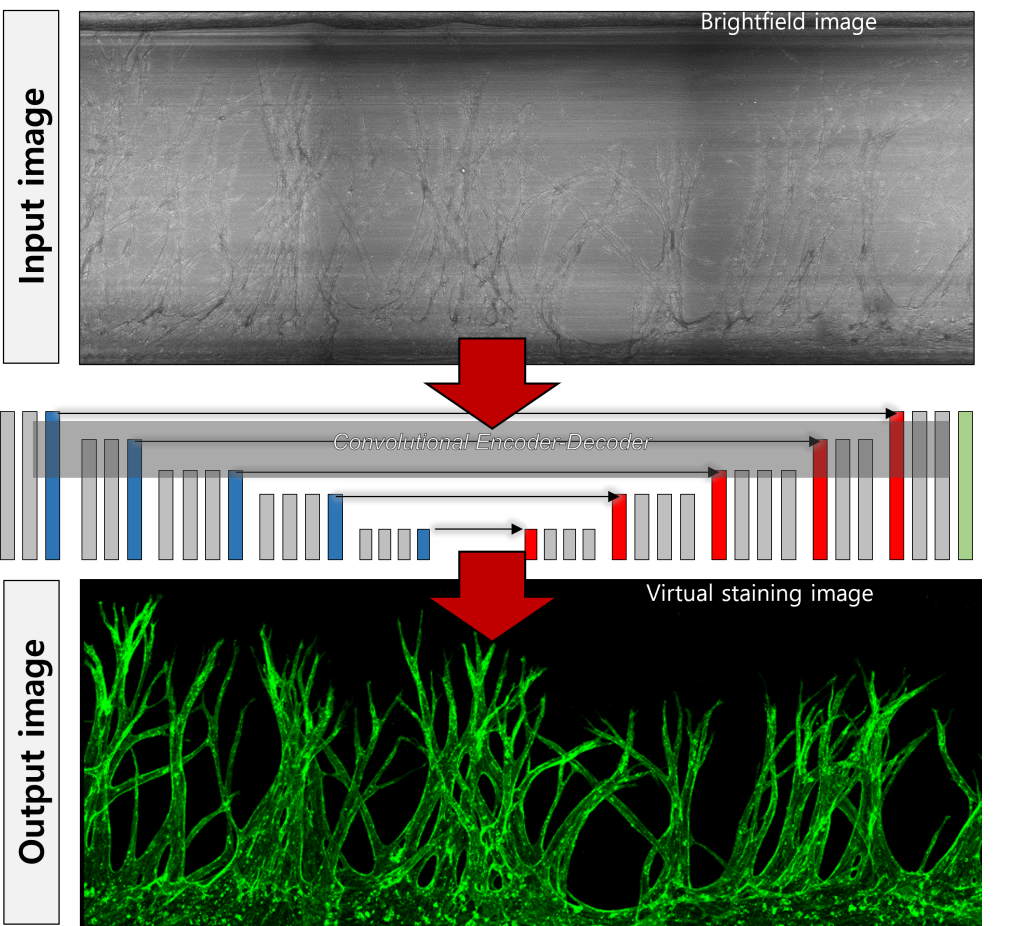
Our research lab is pioneering computer vision techniques to transform the analysis and visualization of biological structures. By integrating deep learning, we uncover crucial quantitative data and enable real-time tracking. Virtual staining allows continuous observation and analysis of cellular processes over time, capturing both temporal and spatial data. We extract quantitative parameters to create comprehensive datasets, which we analyze to uncover critical insights and patterns. Live-cell imaging without cell fixation facilitates dynamic analysis of cell migration and morphological changes. Additionally, 3D image reconstruction from multi-focus 2D images provides detailed visualization of cellular structures.
“Angio-Net: deep learning-based label-free detection and morphometric analysis of in vitro angiogenesis”
Suryong Kim, Jungseub Lee, Jihoon Ko, Seonghyuk Park, Seung-Ryeol Lee, Youngtaek Kim, Taeseung Lee, Sunbeen Choi, Jiho Kim, Wonbae Kim, Yoojin Chung, Oh-Heum Kwond and Noo Li Jeon, Lab Chip 24, 751-763 (2024)
“Machine Learning-Aided Three-Dimensional Morphological Quantification of Angiogenic Vasculature in the Multiculture Microfluidic Platform”
Wonjun Lee, Byoungkwon Yoon, Jungseub Lee, Sangmin Jung, Young Sun Oh, Jihoon Ko & Noo Li Jeon, BioChip J, 17, 357–368 (2023)
Genomics
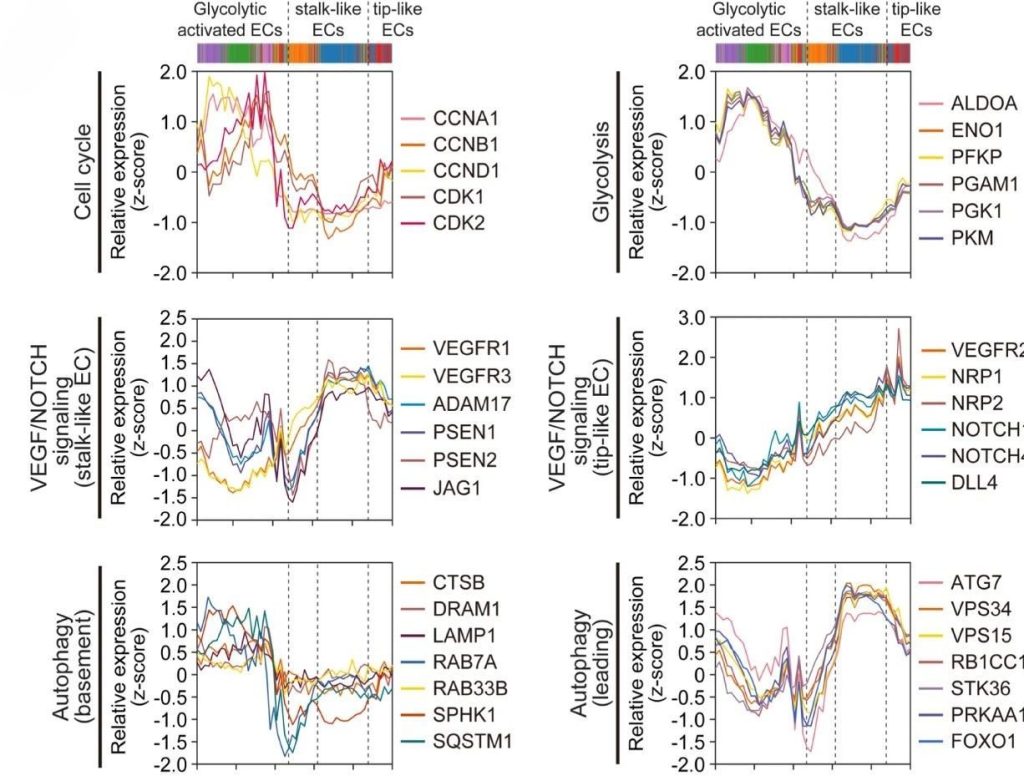
Gene expression analysis is vital for identifying disease causes, developing diagnostic markers, creating treatments, predicting drug responses, and conducting basic research. It detects changes in gene expression patterns and helps design personalized therapies using methods like RNA-Seq, qPCR, microarray analysis, single-cell RNA-Seq, and bioinformatics. Genomic analysis with organ-on-a-chip technology simulates the human physiological environment more accurately than traditional systems, mimicking the 3D structure and fluid flow of the human body. This technology allows precise microenvironment control and high-throughput screening, improving research efficiency, reducing ethical concerns, and lowering costs.
“Angiogenesis-on-a-chip coupled with single-cell RNA sequencing reveals spatially differential activations of autophagy along angiogenic sprouts”
Somin Lee, Hyunkyung Kim, Bum Suk Kim, Sehyun Chae, Sangmin Jung, Jung Seub Lee, James Yu, Kyungmin Son, Minhwan Chung, Jong Kyong Kim, Daehee Hwang, Sung Hee Baek, Noo Li Jeon, Nature Communications, 15 (2024)
“3D Microfluidic Platform and Tumor Vascular Mapping for Evaluating Anti-Angiogenic RNAi-Based Nanomedicine”
Somin Lee, Seongchan Kim, Dong-Jun Koo, James Yu, Hyeongjun Cho, Hyojin Lee, Joon Myong Song, Sung-Yon Kim, Dal-Hee Min, Noo Li Jeon, ACS nano, (2021)
“High-throughput chemical screening to discover new modulators of microRNA expression in living cells by using graphene-based biosensor”
Soo-Ryoon Ryoo, Yeajee Yim, Young-Kwan Kim, Il-Soo Park, Hee-Kyung Na, Jieon Lee, Hongje Jang, Cheolhee Won, Sungwoo Hong, Sung-Yon Kim, Noo Li Jeon, Joon Myong Song & Dal-Hee Min, Scientific reports, 8 (1), 11413(2018)
Next Generation Pathology
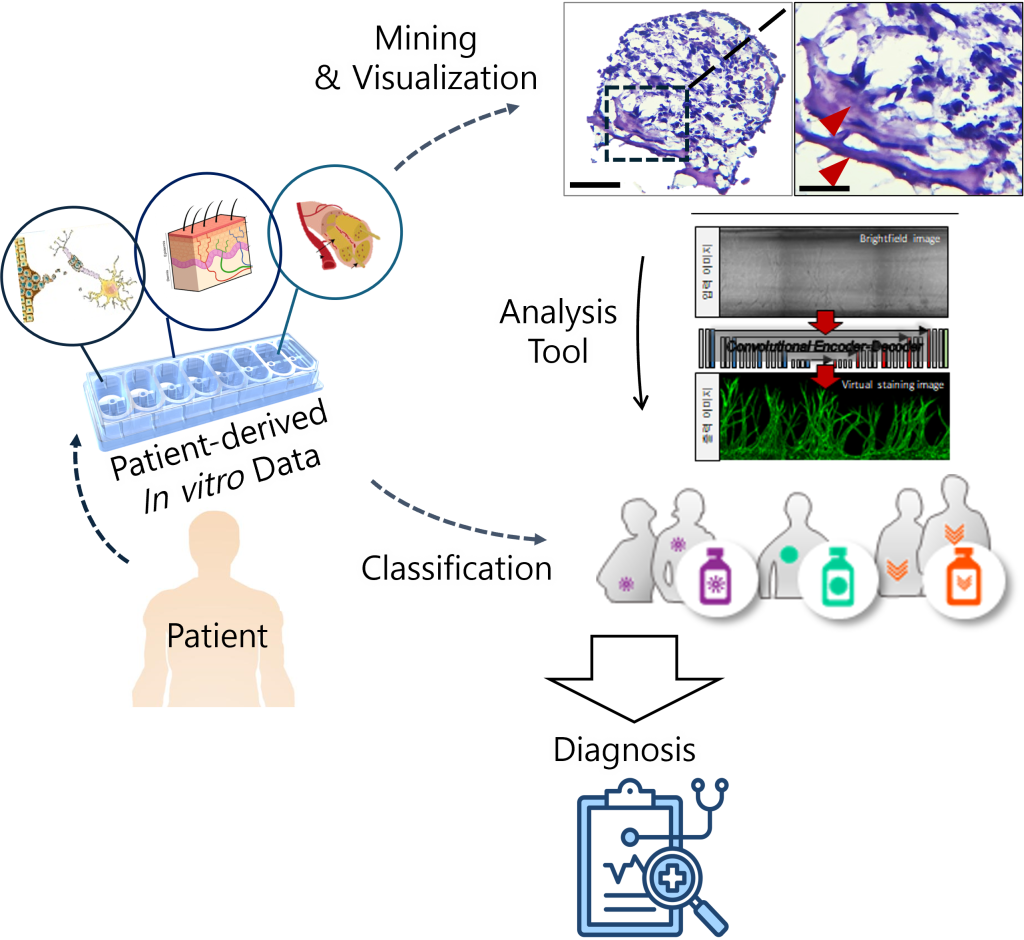
Next Generation Pathology (NGP) uses advanced technologies like digital pathology, AI, and 3D tissue modeling to revolutionize diagnosis, research, and treatment in pathology. Digital pathology enables remote analysis, while AI improves diagnostic accuracy by analyzing large datasets. Genomics and molecular pathology facilitate personalized treatments by studying diseases at the genetic level. Organ-on-a-chip technology recreates human organ environments for detailed disease studies and drug development. Combining NGP with organ-on-a-chip allows precise disease modeling, personalized treatments, and cost-effective, high-resolution data, advancing pathology and healthcare.
“Next-Generation Pathology”
Peter D Caie, David J Harrison, Methods Mol Biol, 1386:61-72(2016)
“Digital pathology and artificial intelligence in translational medicine and clinical practice “
Vipul Baxi, Robin Edwards, Michael Montalt and Saurabh Saha, Modern Pathology, 35:23 – 32(2022)
” Introduction to Digital Image Analysis in Whole-slide imaging: A White Paper from the Digital Pathology Association”
Famke Aeffner, Mark D. Zarella, Nathan Buchbinder, Marilyn M. Bui, Matthew R. Goodman, Douglas J. Hartman, Giovanni M. Lujan, Mariam A. Molani, Anil V. Parwani, Kate Lillard, Oliver C. Turner, Venkata N.P. Vemuri, Ana G. Yuil-Valdes, Douglas Bowman, J Pathol Inform, 1:9 (2019)